
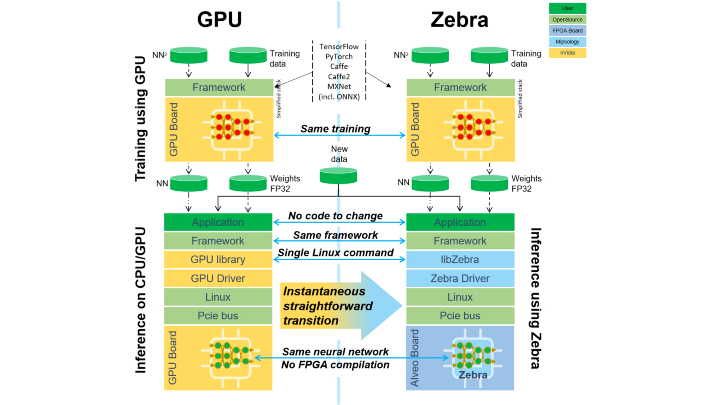
Thanks to their many thousand cores, the graphics processing units are better at machine learning than the central processing units. A good graphics card will make sure the computation of neural networks goes well. It’s important for machine learning to have a good graphics processing unit. Is graphics card necessary for machine learning? If you want to go further with a more powerful graphics card, I would recommend that you have access to a more powerful one. Those with larger models might not be able to train them because of the small batches required. The best performance/price ratio for deep learning is offered by the RTX 3080 graphics card. The best value graphics card on the market for deep learning is the RTX 3090, which can be found at a fraction of the cost of other graphics cards. The Graphics Processing Unit (GPU) of the NVIDIA RTX 3090 was faster than all of the other units.
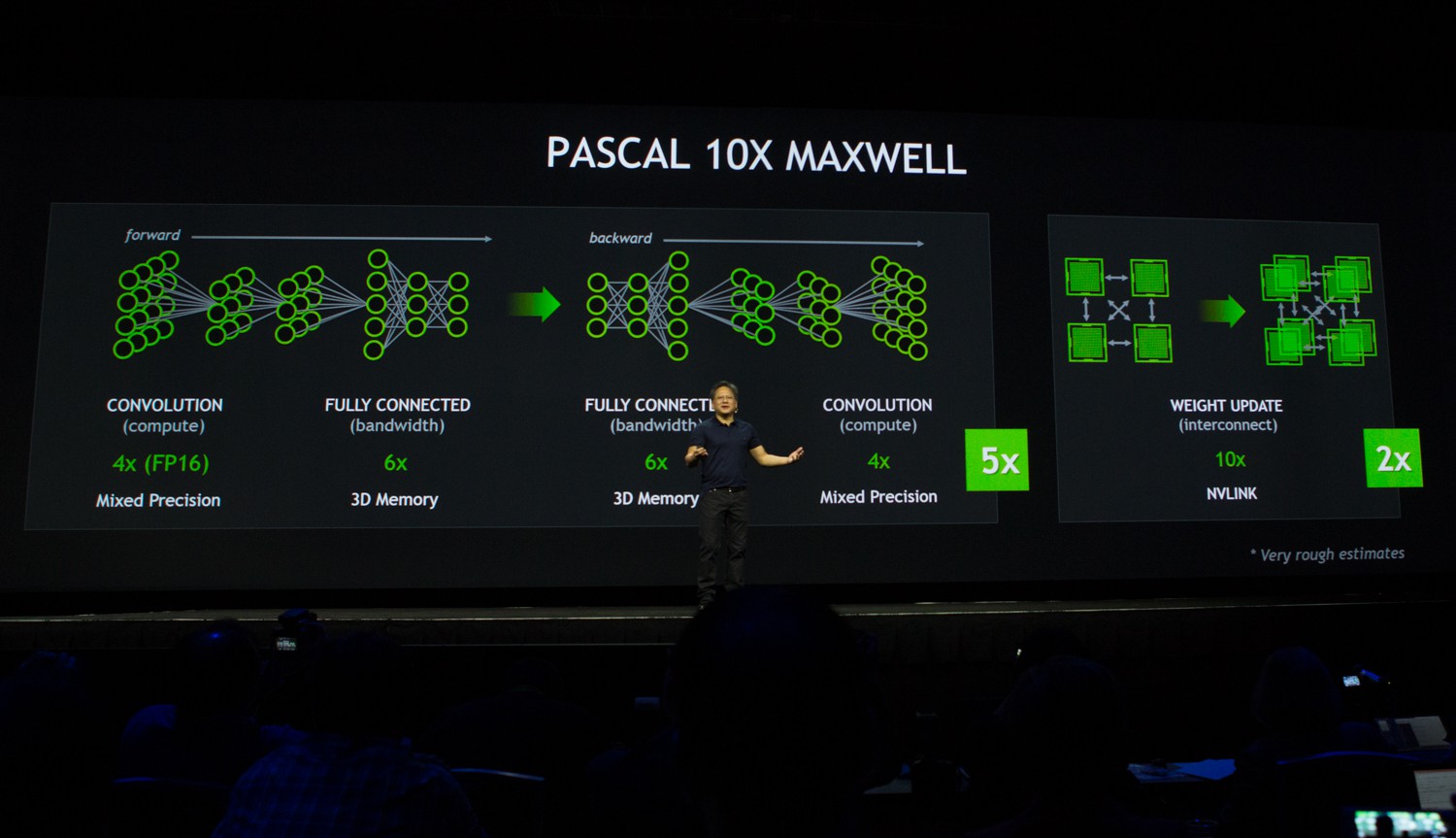
tensorflow isn’t written in that, so you need to use OPENCL for it to work, and it can’t run on any of the AMD graphics cards. It’s possible to run tensorflow on a graphics card, but it would be a huge problem. In order to improve game performance and image quality, the deep learning neural network processing techniques used in the Tensor cores in the RTX graphics card can be used. It has been revealed that the RTX 2080 Ti is twice as fast as the GTX1080 Ti. They can perform tasks with large cache of data and multiple parallel computations in a fraction of the time it takes with non-optimized software. The parallel processing capability of a graphics card makes it much faster than a computer. If you want to work with image data set or training a Convolution neural network, you need at least 4 gigabytes of RAM and 2 gigabytes of graphics card. Is 2GB graphics card enough for machine learning? There was a 20-fold performance enhancement using the board. The matrix multiplication of a neural network can be used to improve the performance of a text detection system. An artificial neural network uses a graphics processing unit.
